TR2008-065
Boosting Adaptive Linear Weak Classifiers for Online Learning and Tracking
-
- "Boosting Adaptive Linear Weak Classifiers for Online Learning and Tracking", IEEE Conference on Computer Vision and Pattern Recognition (CVPR), June 2008, pp. 1-8.BibTeX TR2008-065 PDF
- @inproceedings{Parag2008jun,
- author = {Parag, T. and Porikli, F. and Elgammai, A.},
- title = {Boosting Adaptive Linear Weak Classifiers for Online Learning and Tracking},
- booktitle = {IEEE Conference on Computer Vision and Pattern Recognition (CVPR)},
- year = 2008,
- pages = {1--8},
- month = jun,
- issn = {1063-6919},
- url = {https://www.merl.com/publications/TR2008-065}
- }
,
- "Boosting Adaptive Linear Weak Classifiers for Online Learning and Tracking", IEEE Conference on Computer Vision and Pattern Recognition (CVPR), June 2008, pp. 1-8.
-
Research Areas:
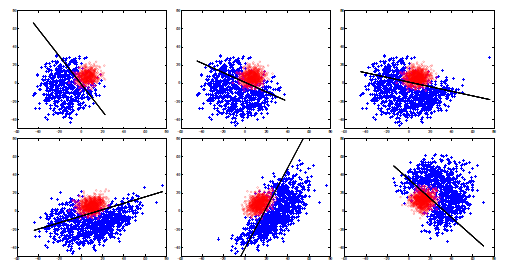
Abstract:
Online boosting methods have recently been used successfully for tracking, background subtraction etc. Conventional online boosting algorithms emphasize on interchanging new weak classifiers/features to adapt with the change over time. We are proposing a new online boosting algorithm where the form of the weak classifiers themselves are modified to cope with scene changes. Instead of replacement, the parameters of the weak classifiers are altered in accordance with the new data subset presented to the online boosting process at each time step. Thus we may avoid altogether the issue of how many weak classifiers to be replaced to capture the change in the data or which efficient search algorithm to use for a fast retrieval of weak classifiers. A computationally efficient method has been used in this paper for the adaptation of linear weak classifiers. The proposed algorithm has been implemented to be used both as an online learning and a tracking method. We show quantitative and qualitative results on both UCI datasets and several video sequences to demonstrate improved performance of our algorithm.
Related News & Events
-
NEWS CVPR 2008: 8 publications by Jay Thornton, Shantanu D. Rane, Oncel Tuzel, Matthew Brand, Anthony Vetro and Amit K. Agrawal Date: June 28, 2008
Where: IEEE Conference on Computer Vision and Pattern Recognition (CVPR)
MERL Contacts: Matthew Brand; Anthony VetroBrief- The papers "Non-Refractive Modulators for Encoding and Capturing Scene Appearance and Depth" by Veeraraghavan, A., Agrawal, A., Raskar, R., Mohan, A. and Tumblin, J., "Feature Transformation of Biometric Templates for Secure Biometric Systems based on Error Correcting Codes" by Sutcu, Y., Rane, S., Yedidia, J.S., Draper, S.C. and Vetro, A., "Constant Time O(1) Bilateral Filtering" by Porikli, F., "Learning on Lie Groups for Invariant Detection and Tracking" by Tuzel, O., Porikli, F. and Meer, P., "Kernel Integral Images: A Framework for Fast non-Uniform Filtering" by Hussein, M., Porikli, F. and Davis, L., "A Conditional Random Field for Automatic Photo Editing" by Brand, M. and Pletscher, P., "Boosting Adaptive Linear Weak Classifiers for Online Learning and Tracking" by Parag, T., Porikli, F. and Elgammai, A. and "Sensing Increased Image Resolution Using Aperture Masks" by Mohan, A., Huang, X., Tumblin, J. and Raskar, R. were presented at the IEEE Conference on Computer Vision and Pattern Recognition (CVPR).