TR2008-010
Joint Tracking and Video Registration by Factorial Hidden Markov Models
-
- "Joint Tracking and Video Registration by Factorial Hidden Markov Models", IEEE International Conference on Acoustics, Speech, and Signal Processing (ICASSP), March 2008, pp. 973-976.BibTeX TR2008-010 PDF
- @inproceedings{Mei2008mar,
- author = {Mei, X. and Porikli, F.},
- title = {{Joint Tracking and Video Registration by Factorial Hidden Markov Models}},
- booktitle = {IEEE International Conference on Acoustics, Speech, and Signal Processing (ICASSP)},
- year = 2008,
- pages = {973--976},
- month = mar,
- issn = {1520-6149},
- url = {https://www.merl.com/publications/TR2008-010}
- }
,
- "Joint Tracking and Video Registration by Factorial Hidden Markov Models", IEEE International Conference on Acoustics, Speech, and Signal Processing (ICASSP), March 2008, pp. 973-976.
-
Research Area:
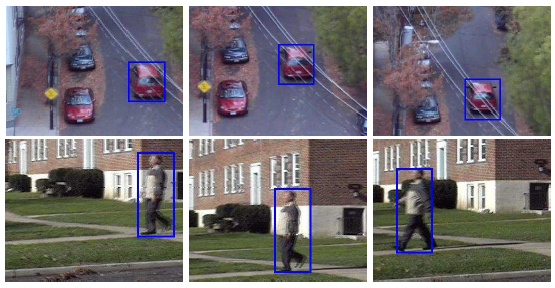
Abstract:
Tracking moving objects from image sequences obtained by a moving camera is a difficult problem since there exists apparent motion of the static background. It becomes more difficult when the camera motion between the consecutive frames is very large. Traditionally, registration is applied before tracking to compensate for the camera motion using parametric motion models. At the same time, tracking result highly depends on the performance of registration. This raises problems when there are big moving objects in the scene and the registration algorithm is prone to fail, since the tracker easily drifts away when poor registration results occur. In this paper, we tackle this problem by registering the frames and tracking the moving objects simultaneously within the factorial Hidden Markov Model framework using particle filters. Under this framework, tracking and registration are not working separately, but mutually benefit each other by interacting. Particles are drawn to provide the candidate geometric transformation parameters and moving object parameters. Background is registered according to the geometric transformation parameters by maximizing a joint gradient function. A state-of-the-art covariance tracker is used to track the moving object. The tracking score is obtained by incorporating both background and foreground information. By using knowledge of the position of the moving objects, we avoid blindly registering the image pairs without taking the moving object regions into account. We apply our algorithm to moving object tracking on numerous image sequences with camera motion and show the robustness and effectiveness of our method.
Related News & Events
-
NEWS ICASSP 2008: 4 publications by MERL researchers and others Date: March 31, 2008
Where: IEEE International Conference on Acoustics, Speech, and Signal Processing (ICASSP)Brief- The papers "Joint Tracking and Video Registration by Factorial Hidden Markov Models" by Mei, X. and Porikli, F., "Speech Denoising Using Nonnegative Matrix Factorization with Priors" by Wilson, K.W., Raj, B., Smaragdis, P. and Divakaran, A., "Ultrasonic Doppler Sensor for Speaker Recognition" by Kalgaonkar, K. and Raj, B. and "Sparse and Shift-Invariant Feature Extraction from Non-Negative Data" by Smaragdis, P., Raj, B. and Shashanka, M. were presented at the IEEE International Conference on Acoustics, Speech, and Signal Processing (ICASSP).